Our Oxidative Stress Doc set is ~41% complete, while our O-linked Glycosylation Disorders Doc set is ~80% complete! Please continue to contribute to the completion of these doc sets, and invite your friends to join us so we can finish this faster!
Thanks to our Mark2Curators, three of our doc sets have already been completed, and we’re currently investigating methods for creating interactive visuals so that the Mark2Cure community can further engage with the work they’ve done. We’ve previously posted some overall stats on each doc set, and would like to take the opportunity to further detail the importance of your contributions.
In the lorenz chart below, you can see how the work for each doc set and for all 3 groups is distributed among our Mark2Curators. If each Mark2Curators contributed the same amount of work, we would expect that the curve would be a straight, diagonal line from (0,0) to (100,100). If only one user completed all the work, you would expect a right angle with a vertex at (0,100). For the smaller doc set, the work was a little more evenly distributed among the contributing Mark2Curators. Overall (and especially for the CDG doc set), our active Mark2Curators completed about 70% of the work, while Mark2Curators who submitted just a few quests were critically important for the completion of the CDG doc set.
For more information about the distribution of effort in citizen science, check out Brooke Simmon’s post at Zooniverse on citizen science metrics for success.
We’ve previously posted some preliminary networks generated from the information Mark2Curators extracted in the various doc sets, but some users have been wondering, how exactly does that compare with the information extracted by machine algorithms and more importantly, why do your contributions matter?
Figure 1 is a network by concepts identified by a machine algorithm, while Figure 2 is a network of concepts identified by Mark2Curators. While both the algorithm and Mark2Cure extract diseases and genes concepts, the algorithm searches for chemical entities. In contrast, Mark2Curators are asked to look for treatments. The machine algorithm also searches for ‘species’ entities, while Mark2Curators do not. In the FBX-ENGASE doc set, the algorithm may be too generous in extracting information, while Mark2Curators are more conservative. By merging the two together (Figure 3) we can build a richer picture of the information embedded in the FBX-ENGASE doc set.
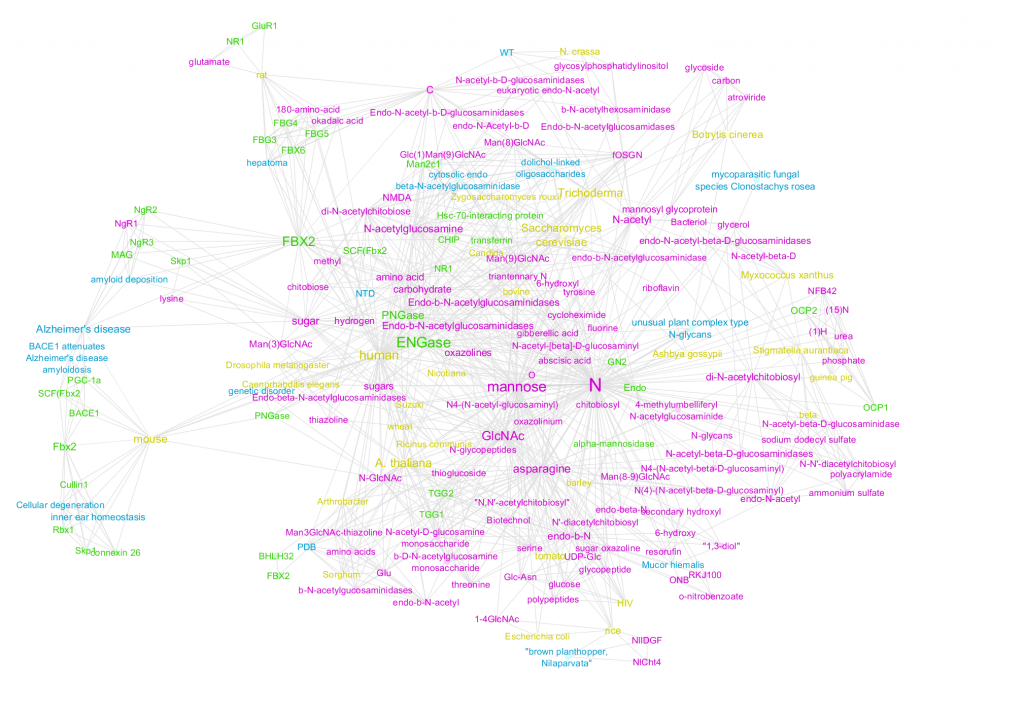
Figure 1 – Disease (blue), chemical (pink), gene (green), and species (yellow) entities extracted by a machine algorithm